We’ve published a Python Script that uses the clustering method to group keywords together using Google’s search results. The new version is improved, and some cool graphical outputs are added to visualize keyword topics more clearly.
Why should you use this python script?
Today, it’s advantageous to optimize full keyword topics rather than single search terms. A big question is how best to structure your content. Sharing the Topic Graph Output with your creators may already be enough briefing to create great content.
Why using the SERP (Search Engine Result Page) results for grouping the keyword topics?
Google focuses a lot on how ranking pages cover the topic. You’ll get instant clustering results. We use graph-based clustering algorithms that use associations of different keywords to rank URLs. Instead of building own cluster logics, we use the shortcut of using googles ranking blackbox.
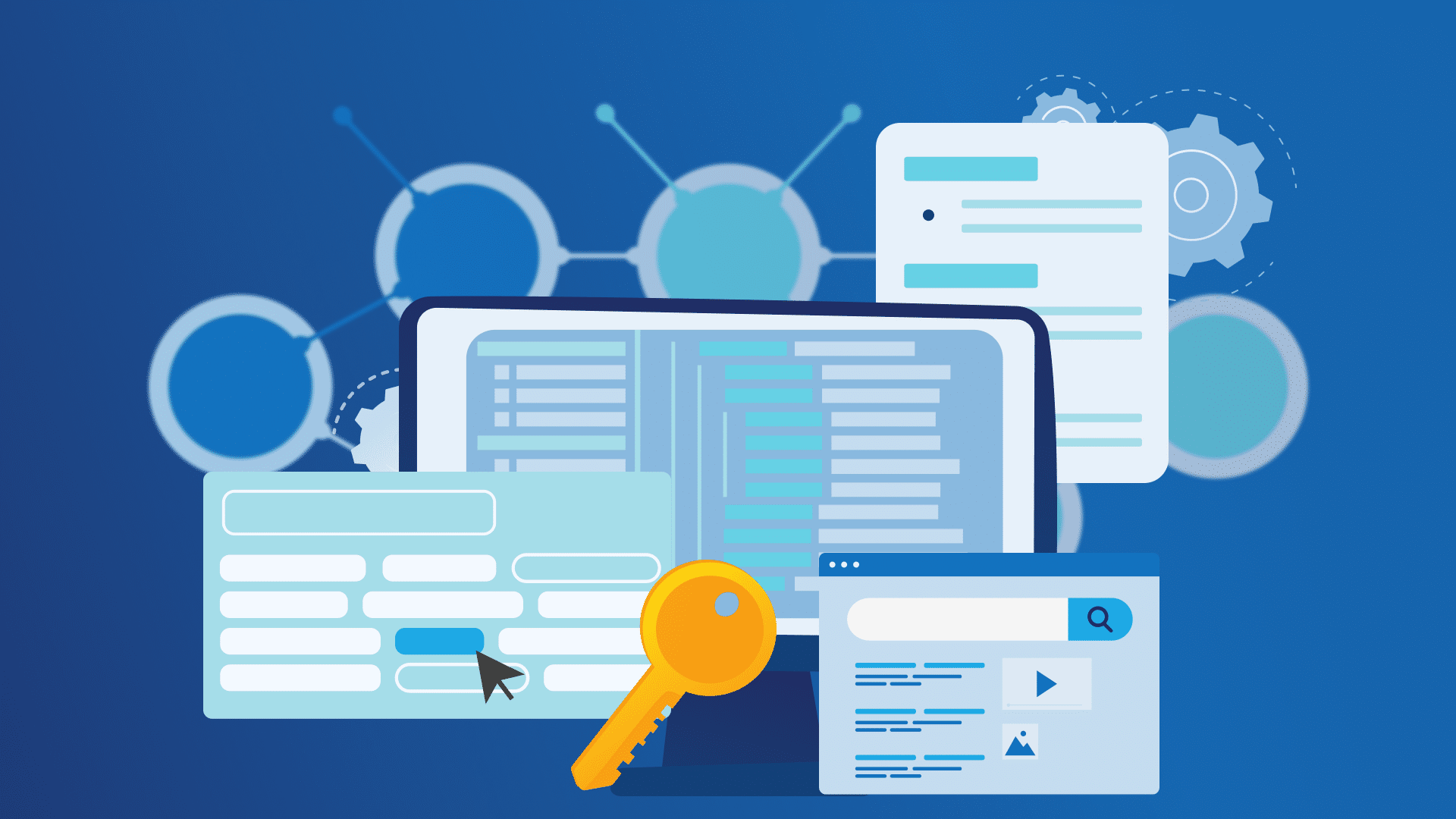
Step 1: Your starter keyword set
We used Google’s keyword planner as a starting point. Of course, you can use any other sources, such as Semrush and Ahrefs. Our seed keyword was “SEO Tool”, and we used the first 100 keywords as input for SERP clustering. Put all the keywords in a list that you think should be part of your keyword topic. This list is clustered by using ranking pages.
Step 2: Run the Python script
The script uses Google Custom Search Engine to fetch the top 10 ranking pages for each keyword. When the Script is finished, you’ll find an image in your script folder that looks like this:
Step 3: Refine input keyword list
You’ll probably notice that some topics are more interesting than others when creating the first graphic output. Get rid of noisy keywords and rerun the algorithm for even better results.
The output generated for our real-world examples was great for structuring the big topic into subtopics in no time. From this point on, it’s up to you to create one large holistic content page that covers subtopics in separate paragraphs or to use multiple linked pages.
Here you can find the Python Script:
from googleapiclient.discovery import build import pandas as pd import Levenshtein from datetime import datetime from fuzzywuzzy import fuzz from urllib.parse import urlparse from tld import get_tld import langid import json import numpy as np import networkx as nx from networkx.algorithms import community import sqlite3 import math import io from collections import defaultdict import matplotlib.pyplot as plt from matplotlib import cm import random def language_detection(str_lan): lan=langid.classify(str_lan) return lan[0] def extract_mainDomain(url): res = get_tld(url, as_object=True) return res.fld def fuzzy_ratio(str1,str2): return fuzz.ratio(str1,str2) def fuzzy_token_set_ratio(str1,str2): return fuzz.token_set_ratio(str1,str2) def google_search(search_term, api_key, cse_id,hl,gl, **kwargs): try: service = build("customsearch", "v1", developerKey=api_key,cache_discovery=False) res = service.cse().list(q=search_term,hl=hl,gl=gl,fields='queries(request(totalResults,searchTerms,hl,gl)),items(title,displayLink,link,snippet)',num=10, cx=cse_id, **kwargs).execute() return res except Exception as e: print(e) return(e) def google_search_default_language(search_term, api_key, cse_id,gl, **kwargs): try: service = build("customsearch", "v1", developerKey=api_key,cache_discovery=False) res = service.cse().list(q=search_term,gl=gl,fields='queries(request(totalResults,searchTerms,hl,gl)),items(title,displayLink,link,snippet)',num=10, cx=cse_id, **kwargs).execute() return res except Exception as e: print(e) return(e) def com_postion(size,scale=1, center=(0, 0), dim=2): # generat the postion for each community num = size center = np.asarray(center) theta = np.linspace(0, 1, num+1)[:-1] * 2 * np.pi theta = theta.astype(np.float32) pos = np.column_stack([np.cos(theta), np.sin(theta), np.zeros((num, 0))]) pos = scale * pos + center return pos def node_postion(one_com,scale=1, center=(0, 0), dim=2): # generat the postion for each nodes in a community num = len(one_com) node = list(one_com) center = np.asarray(center) theta = np.linspace(0, 1, num+1)[:-1] * 2 * np.pi theta = theta.astype(np.float32) pos = np.column_stack([np.cos(theta), np.sin(theta), np.zeros((num, 0))]) pos = scale * pos + center pos = dict(zip(node, pos)) return pos def getClustersWithPlotting(DATABASE,SERP_TABLE,CLUSTER_TABLE,TIMESTAMP="max"): dateTimeObj = datetime.now() options = {'font_family': 'serif', 'font_size': '12', 'font_color': '#000000'} connection = sqlite3.connect(DATABASE) if TIMESTAMP=="max": df = pd.read_sql(f'select * from {SERP_TABLE} where requestTimestamp=(select max(requestTimestamp) from {SERP_TABLE})', connection) else: df = pd.read_sql(f'select * from {SERP_TABLE} where requestTimestamp="{TIMESTAMP}"', connection) G = nx.Graph() #add graph nodes from dataframe columun G.add_nodes_from(df['searchTerms']) #add edges between graph nodes: for index, row in df.iterrows(): df_link=df[df["link"]==row["link"]] for index1, row1 in df_link.iterrows(): G.add_edge(row["searchTerms"], row1['searchTerms']) # community detection com = community.greedy_modularity_communities(G) num_com = len(com) clusters_list=[] for val in range(num_com): clusters_list.append([dateTimeObj,val,' | '.join(list(com[val]))]) df_clusters=pd.DataFrame(clusters_list,columns=["requestTimestamp","cluster","searchTerms"]) #save to sqlite cluster table connection = sqlite3.connect(DATABASE) df_clusters.to_sql(name=CLUSTER_TABLE,index=False,if_exists="append",dtype={"requestTimestamp": "DateTime"}, con=connection) # find intra_com links intra_links = {} for i in range(num_com): intra_links[i] = [] for link in nx.edges(G): for i in range(num_com): if (link[0] in com[i]) & (link[1] in com[i]): intra_links[i].append(link) com_center = com_postion(num_com, scale=3) # print(com_center) pos = dict() for val in range(num_com): node_pos = node_postion(com[val], scale=0.8, center=com_center[val]) pos.update(node_pos) plt.figure(figsize=(25,15), dpi=150) nx.draw(G, pos, with_labels=True, edgelist=[], **options,node_size=10) nx.draw_networkx_edges(G, pos, alpha=0.08, width=0.5,node_size=10) #degree for each node will be used for node size d = dict(G.degree) #creat list of random colors same number as communities number colors = ["#"+''.join([random.choice('0123456789ABCDEF') for j in range(6)]) for i in range(num_com)] for val in range(num_com): nx.draw_networkx_nodes(G, pos, node_size=[d[v] * 10 for v in list(com[val])], nodelist=list(com[val]), node_color=colors[val]) nx.draw_networkx_edges(G, pos, alpha=0.1, edgelist=intra_links[val], width=1.5) plt.axis("off") plt.savefig('keyword_community.png', format='png', dpi=150) plt.show() def getSearchResult(filename,hl,gl,my_api_key,my_cse_id,DATABASE,TABLE): dateTimeObj = datetime.now() rows_to_insert=[] keyword_df=pd.read_csv(filename) keywords=keyword_df.iloc[:,0].tolist() for query in keywords: if hl=="default": result = google_search_default_language(query, my_api_key, my_cse_id,gl) else: result = google_search(query, my_api_key, my_cse_id,hl,gl) if "items" in result and "queries" in result : for position in range(0,len(result["items"])): result["items"][position]["position"]=position+1 result["items"][position]["main_domain"]= extract_mainDomain(result["items"][position]["link"]) result["items"][position]["title_matchScore_token"]=fuzzy_token_set_ratio(result["items"][position]["title"],query) result["items"][position]["snippet_matchScore_token"]=fuzzy_token_set_ratio(result["items"][position]["snippet"],query) result["items"][position]["title_matchScore_order"]=fuzzy_ratio(result["items"][position]["title"],query) result["items"][position]["snippet_matchScore_order"]=fuzzy_ratio(result["items"][position]["snippet"],query) result["items"][position]["snipped_language"]=language_detection(result["items"][position]["snippet"]) for position in range(0,len(result["items"])): rows_to_insert.append({"requestTimestamp":dateTimeObj,"searchTerms":query,"gl":gl,"hl":hl, "totalResults":result["queries"]["request"][0]["totalResults"],"link":result["items"][position]["link"], "displayLink":result["items"][position]["displayLink"],"main_domain":result["items"][position]["main_domain"], "position":result["items"][position]["position"],"snippet":result["items"][position]["snippet"], "snipped_language":result["items"][position]["snipped_language"],"snippet_matchScore_order":result["items"][position]["snippet_matchScore_order"], "snippet_matchScore_token":result["items"][position]["snippet_matchScore_token"],"title":result["items"][position]["title"], "title_matchScore_order":result["items"][position]["title_matchScore_order"],"title_matchScore_token":result["items"][position]["title_matchScore_token"], }) df=pd.DataFrame(rows_to_insert) #save serp results to sqlite database connection = sqlite3.connect(DATABASE) df.to_sql(name=TABLE,index=False,if_exists="append",dtype={"requestTimestamp": "DateTime"}, con=connection) ############################################################################################################################################## #Read Me: # ############################################################################################################################################## #1- You need to setup a google custom search engine. # # Please Provide the API Key and the SearchId. # # Also set your country and language where you want to monitor SERP Results. # # If you don't have an API Key and Search Id yet, # # you can follow the steps under Prerequisites section in this page https://developers.google.com/custom-search/v1/overview#prerequisites # # # #2- You need also to enter database, serp table and cluster table names to be used for saving results. # # # #3- enter csv file name or full path that contains keywords that will be used for serp # # # #4- For keywords clustering and plotting enter the timestamp for serp results that will used for clustering. # # If you need to cluster last serp results enter "max" for timestamp. # # or you can enter specific timestamp like "2021-02-18 17:18:05.195321" # # # #5- Browse the results through DB browser for Sqlite program. The clusters plot will save to keyword_community.png # # ############################################################################################################################################## #csv file name that have keywords for serp CSV_FILE="keywords.csv" # determine language LANGUAGE = "en" #detrmine country COUNTRY = "us" #google custom search json api key API_KEY="XXXXXX" #Search engine ID CSE_ID="XXXXXX" #sqlite database name DATABASE="keywords.db" #table name to save serp results to it SERP_TABLE="keywords_serps" # run serp for keywords getSearchResult(CSV_FILE,LANGUAGE,COUNTRY,API_KEY,CSE_ID,DATABASE,SERP_TABLE) #table name that cluster results will save to it. CLUSTER_TABLE="keyword_clusters" #Please enter timestamp, if you want to make clusters and graph for specific timestamp #If you need to make clusters and graph for the last serp result, send it with "max" value #TIMESTAMP="2021-02-18 17:18:05.195321" TIMESTAMP="max" #run keyword clusters according to networks and community algorithms then save them in sqlite and plot keyword communities (keyword_community.png) getClustersWithPlotting(DATABASE,SERP_TABLE,CLUSTER_TABLE,TIMESTAMP)